Description: This article provides general information about the impact of machine learning in finance. For personalized financial advice, please consult a financial expert or a certified professional
In a data-driven world, the fusion of finance and technology has led to significant advances in managing money, predicting outcomes, and securing transactions. As we approach 2025, machine learning in finance is becoming a pivotal force in reshaping the financial industry, providing new solutions for everything from fraud detection to personal finance management.
Machine learning, a branch of artificial intelligence, has been harnessed to transform how financial institutions, businesses, and individuals make decisions. With its ability to process vast amounts of data and extract meaningful insights, machine learning drives innovation across various finance sectors. This article will explore how machine learning transforms finance and what it means for the future.
The Role of Machine Learning in Finance: Why It Matters
Machine learning in finance is not just a trend; it’s a revolution. Financial institutions are now adopting these technologies to enhance operational efficiency, improve customer experiences, and minimize risks. Machine learning provides previously impossible solutions, from automating decision-making processes to predictive analytics.
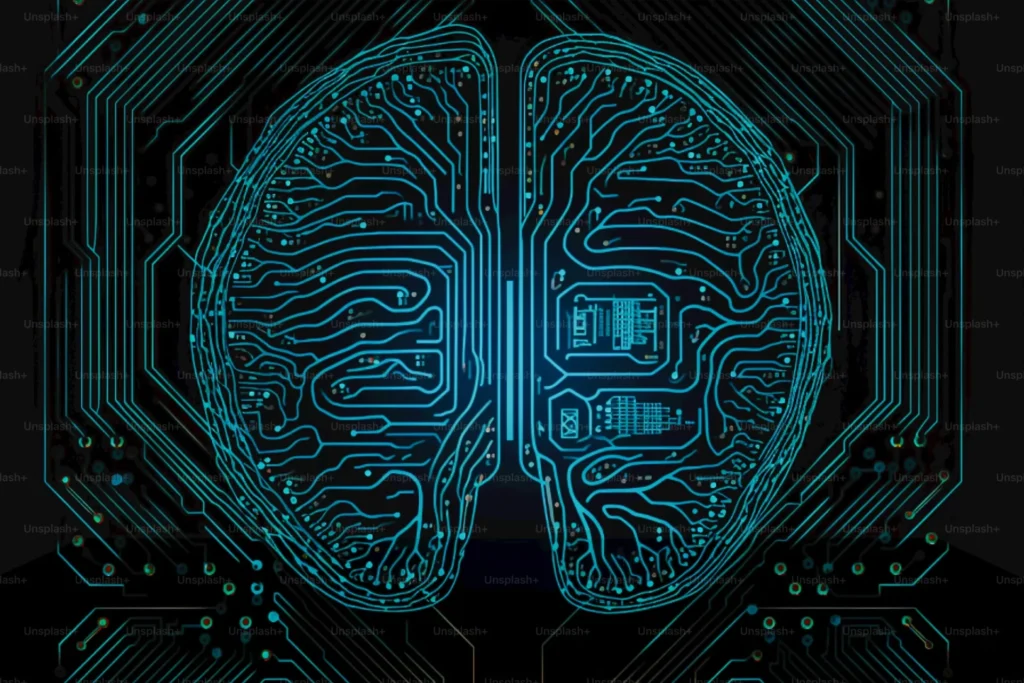
Enhancing Fraud Detection and Prevention
One of the first and most impactful applications of machine learning development services in finance has been fraud detection. Traditional fraud prevention systems rely on rule-based algorithms, which can struggle to adapt to evolving fraudulent tactics. Machine learning, however, uses algorithms that can process massive amounts of transactional data and identify patterns, anomalies, and suspicious activities in real time.
By learning from past fraud cases, machine learning systems continuously improve their detection capabilities, enabling quicker responses to potential threats and reducing the risk of financial loss. Institutions that implement advanced machine learning tools can identify fraudulent transactions faster, minimizing the impact of fraud and shortening investigation times. For instance, McKinsey & Company discusses how financial institutions can use advanced analytics to combat evolving payments fraud while enhancing customer experience.
Automating Credit Scoring: Making Lending Fairer
The credit scoring process has always been one of the most critical aspects of financial transactions, especially in lending. However, traditional credit scoring models are based on a narrow set of criteria, often leading to biases or overlooked financial behaviors. Machine learning changes this using broader data points, including non-traditional factors such as social media activity, utility bill payments, and even behavioral data.
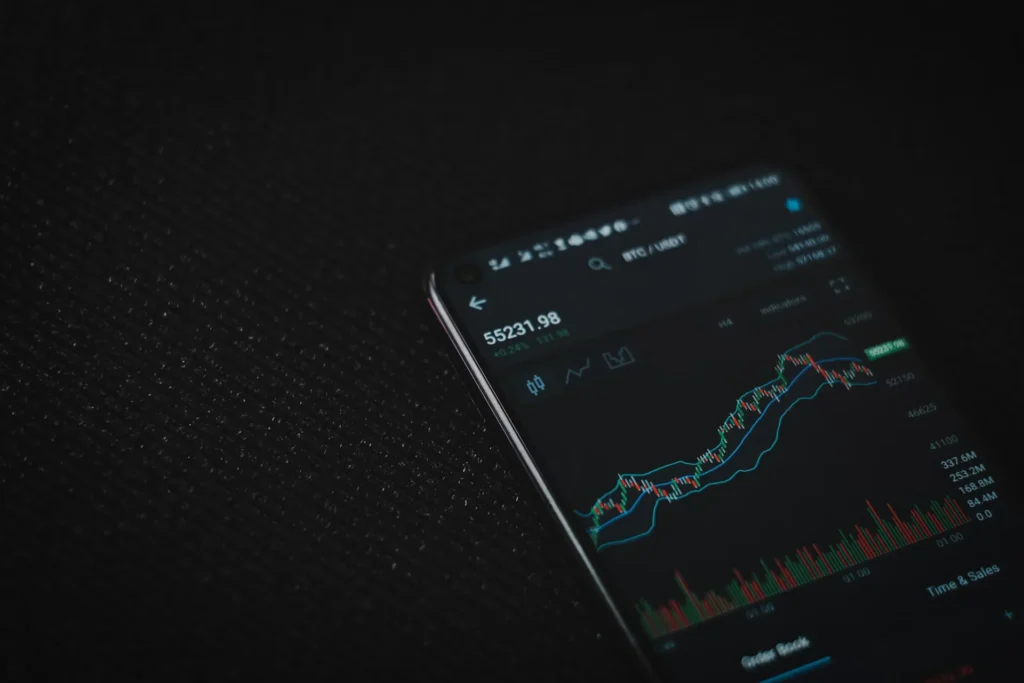
This enables machine learning development services to provide a more holistic, accurate view of an individual’s creditworthiness. As a result, borrowers who traditional systems might have previously overlooked can now gain access to credit, which can significantly improve financial inclusion. Upstart’s official website shares how their machine learning model has been used to increase access to credit for those typically denied by traditional methods.
Personalizing Customer Experiences
Personalization has become one of the key drivers of customer loyalty, and in finance, machine learning is making it easier than ever to offer tailored experiences. Financial institutions now use machine learning to analyze customer behavior, preferences, and transaction histories to provide personalized products and services.
For example, by leveraging predictive analytics, banks can offer customized financial advice, recommend relevant investment options, or even send alerts about upcoming expenses. By processing vast datasets, machine learning allows companies to fine-tune their offerings and deliver precisely what the customer needs, improving satisfaction and engagement. Case studies by TechCrunch show how banks have integrated AI to significantly enhance the personalization of their services, resulting in greater customer retention.
Smarter Investments and Trading
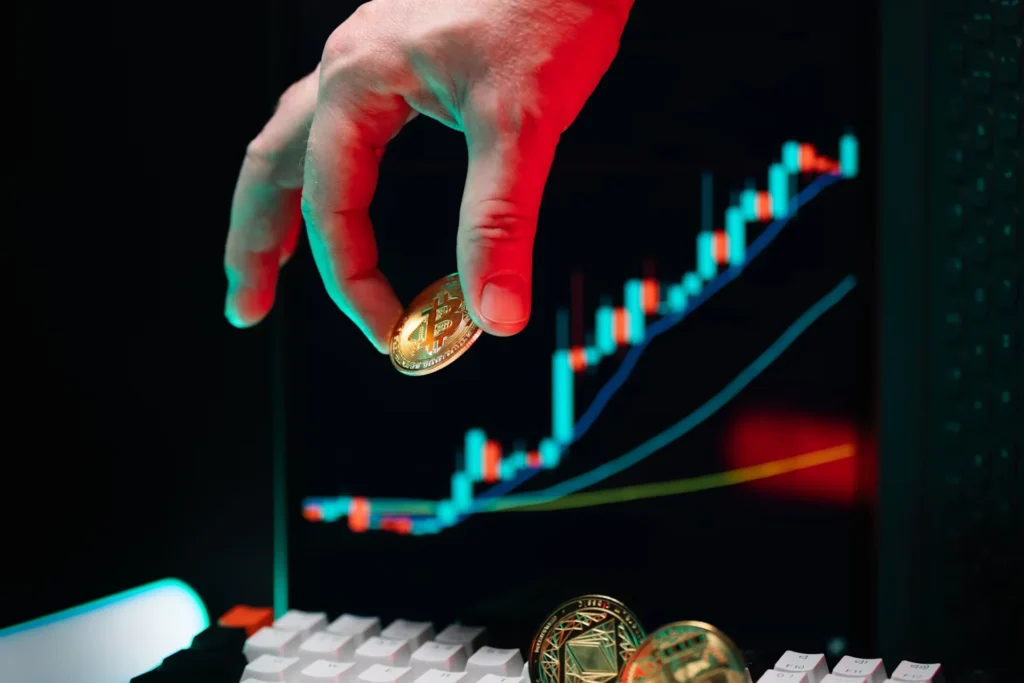
One of the most widely recognized applications of machine learning in finance is in algorithmic trading. Trading algorithms use machine learning to analyze real-time market data, identify patterns, and make decisions at a speed far beyond human capabilities. These systems continuously adapt and improve based on historical data, enabling traders to optimize their strategies and increase their returns.
Forecasting market trends with greater precision can also help investors make better decisions. Machine learning development services are helping hedge funds, asset managers, and individual investors tap into more efficient and data-driven investment strategies, allowing them to manage risk while maximizing profitability. An example is the work being done by Renaissance Technologies, whose success in algorithmic trading is powered by sophisticated machine learning models. Forbes has discussed how hedge funds use AI to optimize investment decisions and improve financial performance.
Optimizing Compliance and Regulation
Compliance is one of the biggest challenges for financial institutions. Ensuring compliance with complex regulations and increasing rules can be time-consuming and costly. Machine learning provides a solution by automating compliance checks and flagging potential risks before they become significant.
For example, machine learning development services can analyze regulatory documents, transaction data, and market activity, ensuring adherence to local and global financial laws. This reduces the risk of fines and legal issues while increasing operational efficiency. PwC discusses how machine learning is used to streamline compliance processes and lower operational costs by automating routine regulatory tasks.
The Future of Machine Learning in Finance: What to Expect by 2025
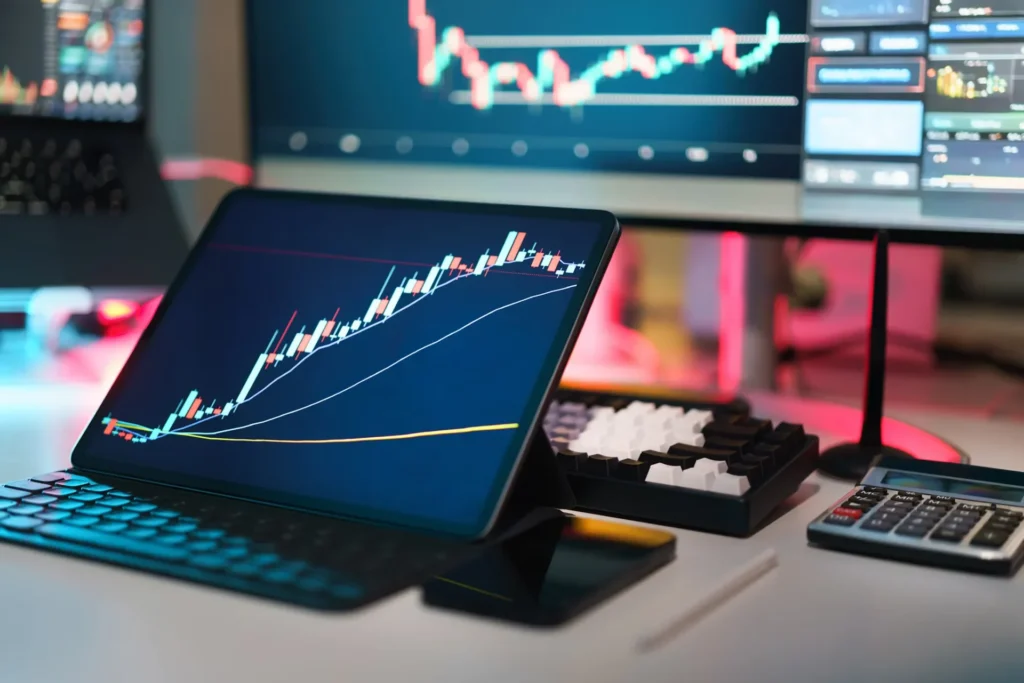
As we look to 2025, the role of machine learning in finance is only expected to grow. Here are a few areas where we can anticipate significant advancements:
- Enhanced Risk Management: Financial institutions will leverage machine learning to predict economic downturns, forecast market movements, and manage risk more effectively.
- Blockchain Integration: Machine learning will work with blockchain technology to provide more secure and transparent financial transactions.
- AI-Driven Personal Finance: Apps and platforms will become even smarter in offering personalized financial planning tools, helping users make more informed decisions about savings, investing, and spending.
Overcoming the Challenges: Ethics, Bias, and Data Privacy
Despite the significant benefits, there are challenges to the widespread adoption of machine learning in finance. One key concern is the risk of algorithm bias, which can perpetuate inequalities. Data privacy is also essential, as financial institutions process vast amounts of sensitive personal information.
As machine learning becomes more ingrained in financial services, these challenges must be addressed by developing more transparent, ethical systems that prioritize fairness and privacy. Future regulations and standards will likely evolve to ensure that the deployment of these technologies is responsible and aligned with societal values.
Conclusion: The Transformative Power of Machine Learning in Finance
Machine learning and AI automation are already transforming the financial landscape, and by 2025, their impact will be even more profound. From personalized customer experiences to more efficient trading and fraud prevention, machine learning and AI automation reshape how the financial industry operates. While challenges such as data privacy and algorithmic bias remain, the benefits of these technologies are undeniable.
By embracing machine learning development services, financial institutions, businesses, and individual investors can unlock a new era of smarter, faster, and more secure financial decision-making. As technology advances, companies must stay ahead by adopting these innovative tools to improve efficiency, reduce risk, and enhance customer satisfaction.